The massive growth of IoT devices and its new applications is driving edge ai with an explosive increase in revenues expected to go from 2.8 billion U.S. dollars in 2019 to 9 billion by 2024. The rise of edge computing has been significantly transforming how organizations are collecting their data, processing it, and gaining insights for more data-driven business decisions. But, what is an edge device?
What are edge devices?
Edge computing is a distributed topology where data storage and processing are done close to the edge devices where it’s being collected, rather than relying on a central location that can be thousands of miles away.
Edge devices are hardware components that control data flow at the boundary between two networks where they serve as network entry (or exit) points.
Enterprises and service providers use edge devices for transmitting, routing, processing, monitoring, filtering, translating, and storing data passing between networks.
Examples of edge devices include cameras, sensors, routers, integrated access devices, multiplexers, and a variety of metropolitan area network and wide area network access devices.
What are benefits of running inferencing on edge devices?
Speed and Latency
Analyzing data, especially real-time data, in edge devices, eliminates latency issues that can affect performance. The less time it takes to analyze data, the more value that comes from it. For example, when it comes to autonomous vehicles, time is of the essence, and most of the data it gathers and processes is useless after a couple of seconds.
Enhanced Security
The distributed architecture that comes with edge computing enables organizations to distribute security risks as well, which diminishes the impact of attacks on the organization as a whole. Edge computing enables organizations to overcome local compliance and privacy regulations’ issues, as well.
Cost Savings
Edge computing helps companies to reduce costs associated with transporting, managing, and securing data. By keeping data within your edge locations, you optimize bandwidth usage to connect all of your locations.
Reliability
Business operations continuity may require local processing of data to avoid possible network outages. Storing and processing data in edge devices improves reliability, and temporary disruptions in network connectivity won’t impact the devices’ operations.
How do edge devices work?
Edge devices have a very simple working principle; it serves as network entry or exit and connects two different networks by translating one protocol into another. Moreover, it creates a secure connection with the cloud.
An edge device is a plug-and-play; its setup is quick and straightforward. It is configured via local access and also has a port to connect it to the internet and the cloud.
How do edge devices offer a better AI experience?
Many Artificial intelligence use-cases are better done on edge devices, offering maximum availability, data security, reduced latency, and optimized costs.
Running Machine learning models can be computationally expensive in cloud-based environments. Meanwhile, inference needs relatively low computing resources. When AI models are trained on the cloud, data needs to be transferred from end-devices to predict outputs. This needs a stable connection, and since the volume of data is large, the transfer can be slow or, in some cases, impossible.
Edge AI moves algorithms closer to the data source where they’re processed locally without requiring any connection offering real-time analytics in less than few milliseconds
The volumes of data are significantly increasing, so is the need to process it autonomously. Enabling Deep Learning algorithms to perform EdgeTraining locally is a must-have feature for many applications such as autonomous vehicles.
IoT edge devices are now able to run machine learning models locally within the device using TensorFlow, Pytorch, or other machine learning tools. The thing that enables capabilities to be handled directly on a device. Localizing the data reduces the latency that results from sending the data to the cloud, and it enables more immediate insights generated by devices.
Massive changes are initiated with Edge AI raising demand for IoT smart devices, and the emergence of more advanced technologies. As organizations are increasingly adopting Edge AI to make their operations better and enable real-time performance, the market will significantly grow to keep pace with the computing requirements of these smart items.
How does Chooch use Edge AI to help businesses?
Chooch Edge AI helps organizations to take their video analytics and IoT applications to the next level. With over 90% of accuracy delivered in less than 0.2 seconds, Chooch provides massive results for many solutions in Artificial Intelligence of Things, manufacturing, fire and smoke detection, healthcare, retail, and more.
Chooch AI creates complete solutions from AI training in the cloud through to deployment. Edge deployments are managed from the cloud, with models that include object recognition, facial authentication, action logging, complex counting, and more.
Currently, Chooch’s Edge AI Vision is able to deploy up to 8 models and 8,000 classes for robust Visual AI on a single edge device. Chooch’s AI inference engines are very fast, generating responses under 0.5 seconds, and processing ten simultaneous calls per second.
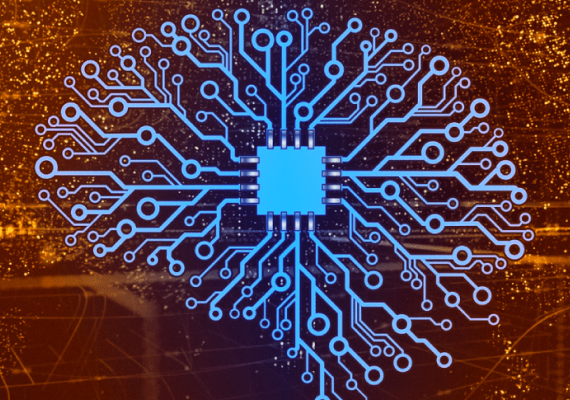
Want to learn more? See how it works.